A good way to show family and friends you care is remembering their birthday. It seems simple enough but in practice, birthday tracking for anyone beyond immediate family and very close friends can be time consuming. Thankfully, you can automate that!
While outsourcing birthday check-in duties does feel a bit impersonal, you can always follow up on the generic message after getting a reply. This post is a tutorial for building a birthday text bot using Twilio.
The first step to building a birthday bot is storing the list of birthdays and contact information somewhere. In this example, I’ve used Coda.io to store name, birthday, and phone number. While I’d prefer to use Google Sheets, Coda’s API interface makes it very easy to import data into the Python environment. Authentication occurs via a bearer token and the API returns a JSON file.
After a bit of unpacking and cleaning, we have a birthday data frame like the one below (this is dummy data, for obvious privacy reasons).
Next, since this code will be deployed to a server and run on a daily schedule, we need to determine which, if any, of our family or friends is celebrating their birthday today.
Finally, we need to tap into the power of Twilio to send the actual SMS message. Twilio is a really cool API service that allows you to programmatically make phone calls and send or receive text messages.
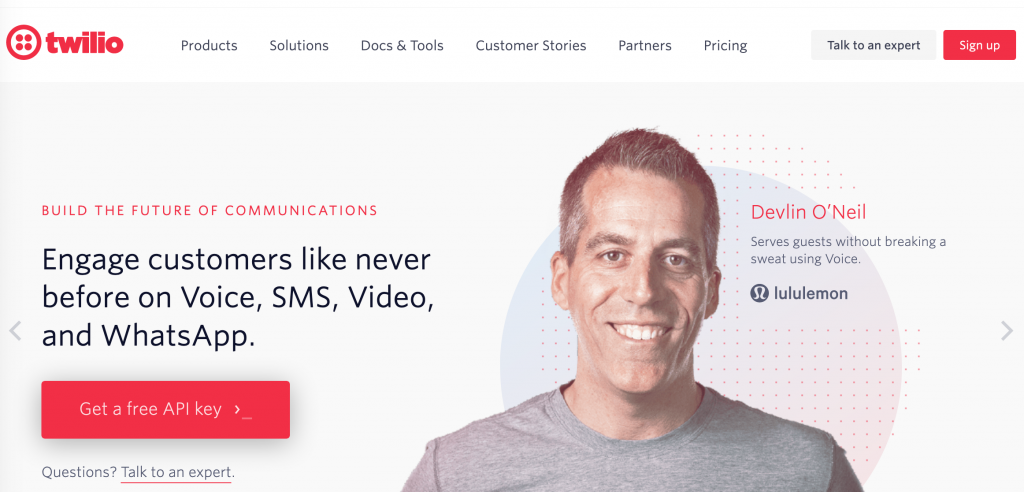
The actual code required to make the birthday bot come to life only requires about eight lines of code. After supplying an account identifier and authentication token, the message client takes as input the body of your text, your Twilio number, and the recipient’s phone number.
That’s it! Let’s see what the message looks like on the recipient’s end.
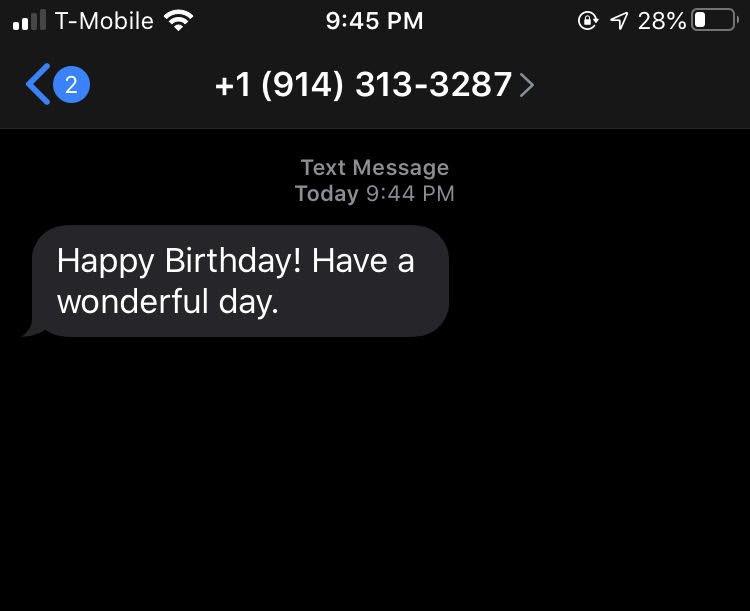
Very slick. By connecting a database (Coda.io) to a messaging API (Twilio), we’ve created a simple birthday text service, capable of earning you the reputation of most thoughtful friend. Enjoy!
Full code can be found on GitHub here.
Feature photo by Sarah Pflug from Burst.